|
ACCESS THE FULL ARTICLE
No SPIE Account? Create one
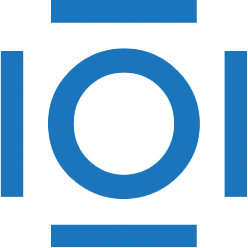
CITATIONS
Cited by 7 scholarly publications.
Damage detection
Feature extraction
Bridges
Continuous wavelet transforms
Wavelets
Convolutional neural networks
Machine learning