|
ACCESS THE FULL ARTICLE
No SPIE Account? Create one
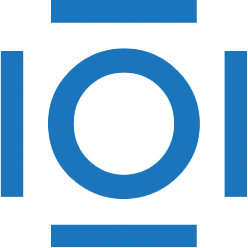
CITATIONS
Cited by 1 scholarly publication.
Evolutionary algorithms
Advanced distributed simulations
Taxonomy
Algorithm development
Artificial intelligence
Statistical analysis
Error analysis