|
ACCESS THE FULL ARTICLE
No SPIE Account? Create one
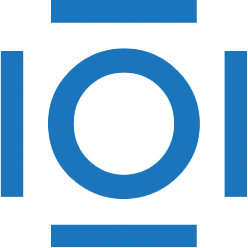
CITATIONS
Cited by 9 scholarly publications.
Image segmentation
Tumors
Breast
Magnetic resonance imaging
Breast cancer
3D image processing
Cancer