|
ACCESS THE FULL ARTICLE
No SPIE Account? Create one
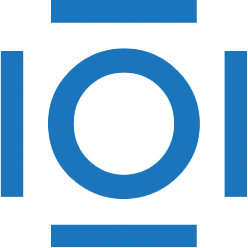
CITATIONS
Cited by 1 scholarly publication.
Adaptive optics optical coherence tomography
Image segmentation
Optical coherence tomography
Retina
Machine learning
Adaptive optics
In vivo imaging