|
ACCESS THE FULL ARTICLE
No SPIE Account? Create one
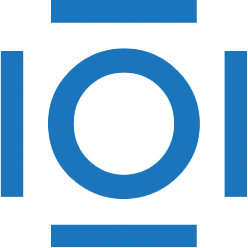
CITATIONS
Cited by 1 scholarly publication.
Autoregressive models
Neural networks
Artificial neural networks
Error analysis
Glasses
Process modeling
Barium