|
ACCESS THE FULL ARTICLE
No SPIE Account? Create one
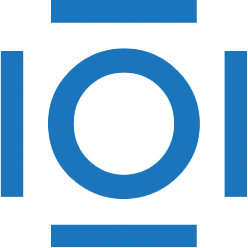
CITATIONS
Cited by 4 scholarly publications.
Neural networks
Land mines
Mining
Digital filtering
Feature extraction
Naval mines
Databases