|
ACCESS THE FULL ARTICLE
No SPIE Account? Create one
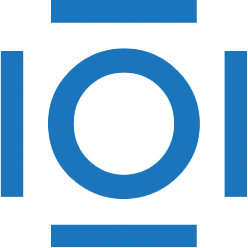
CITATIONS
Cited by 2 scholarly publications.
Earth observing sensors
Landsat
Forestry
Neural networks
Remote sensing
Statistical analysis
Computer simulations