|
ACCESS THE FULL ARTICLE
No SPIE Account? Create one
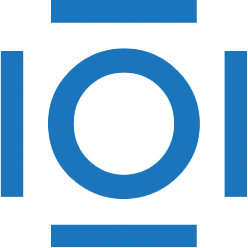
CITATIONS
Cited by 1 scholarly publication.
Neurons
Proteins
Associative arrays
Reliability
Binary data
Data modeling
Data storage