|
ACCESS THE FULL ARTICLE
No SPIE Account? Create one
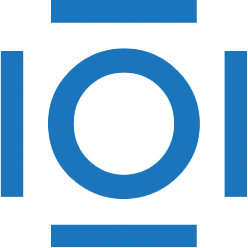
CITATIONS
Cited by 23 scholarly publications.
Visualization
Data mining
Binary data
Databases
Feature selection
Distance measurement
Error analysis