|
ACCESS THE FULL ARTICLE
No SPIE Account? Create one
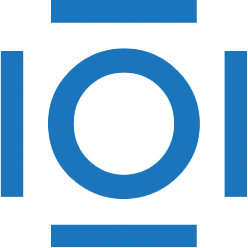
CITATIONS
Cited by 5 scholarly publications.
Image filtering
3D modeling
3D image processing
Bayesian inference
Image analysis
Monte Carlo methods
Amplifiers