|
ACCESS THE FULL ARTICLE
No SPIE Account? Create one
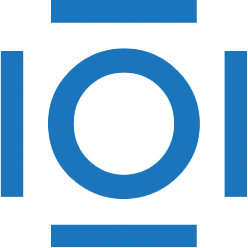
CITATIONS
Cited by 22 scholarly publications.
Particle filters
Particles
Personal digital assistants
Sensors
Filtering (signal processing)
Error analysis
Motion models