|
ACCESS THE FULL ARTICLE
No SPIE Account? Create one
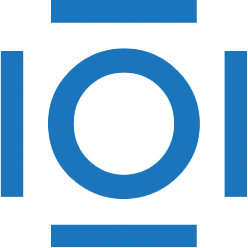
CITATIONS
Cited by 5 scholarly publications and 1 patent.
Neural networks
Principal component analysis
Data modeling
Data acquisition
Etching
Plasma etching
Process modeling