|
ACCESS THE FULL ARTICLE
No SPIE Account? Create one
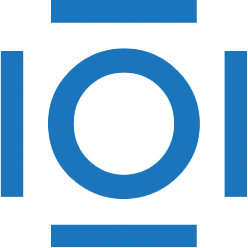
CITATIONS
Cited by 1 scholarly publication.
Shape memory alloys
Buildings
Earthquakes
Optical isolators
Performance modeling
Control systems
Genetic algorithms