|
ACCESS THE FULL ARTICLE
No SPIE Account? Create one
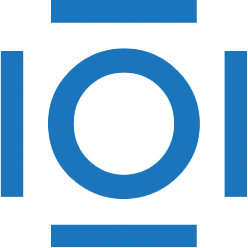
CITATIONS
Cited by 4 scholarly publications.
Principal component analysis
Image classification
Hyperspectral imaging
Spectrometers
Environmental sensing
Imaging systems
Minerals