Polarimetric SAR (PolSAR) can comprehensively describe the differences of targets combining the polarimetric scattering power and the relative phase information among the polarimetric channels. For the traditional image classification methods, a single classifier cannot fully solve the classification problem, in which certain inevitable bias between the classification results and the actual condition exists. To improve the classification performance, ensemble learning theory has been introduced into PolSAR classification, in which a number of different learners are integrated to get the final results. However, a larger number of learners may also lead to some negative effects. The concept of selective ensemble learning (SEL) is then introduced, in which some better learners are selected from a group of individual learners according to the selection strategy and then integrated to obtain a generalized classifier. Based on this idea, a SEL-based PolSAR image classification method is proposed, in which the representative features are extracted based on the characteristics of PolSAR image meanwhile high-performing learners are selected using genetic algorithm optimization. In order to verify the effectiveness and application of the proposed SEL-based PolSAR image classification method, the tests are implemented using UAVSAR L-band PolSAR data, besides the other two newly acquired experimental data of Chinese airborne and spaceborne system. The quantitative and qualitative experimental results confirm that the proposed method has excellent performance in classification. |
ACCESS THE FULL ARTICLE
No SPIE Account? Create one
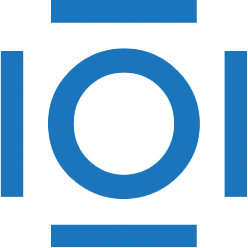
CITATIONS
Cited by 1 scholarly publication.
Image classification
Buildings
Polarimetry
Scattering
Electroluminescence
Feature extraction
Synthetic aperture radar