Ship detection from synthetic aperture radar (SAR) images plays an important role in marine safety and ocean resource management. Unsupervised ship detection methods have a complex set of rules, while supervised methods, such as deep learning approaches, consume substantial time and manpower to make training samples. We demonstrate that ships in an SAR image can be detected by a weakly supervised convolutional neural network that combines new deep learning technology called class activation mapping with the conditional random field. Our model is trained using only SAR images with two global labels, namely, “ship” and “nonship,” and produces three types of output: ship location heatmap, ship bounding box, and pixel-level segmentation product. Experiments on Chinese Gaofen-3 fine strip SAR images validate the effectiveness of the proposed method. Compared with the state-of-the-art methods, our method achieves higher detection accuracy and more intelligent detection characteristics. |
ACCESS THE FULL ARTICLE
No SPIE Account? Create one
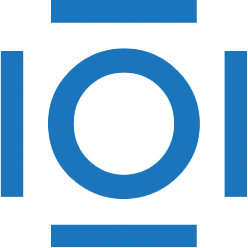
CITATIONS
Cited by 4 scholarly publications.
Synthetic aperture radar
Content addressable memory
Image segmentation
Target detection
Image filtering
Detection and tracking algorithms
Nonlinear filtering