1.IntroductionIn terms of biomedical optical imaging, the biological tissues are conditionally divided into two major groups.1 The first group contains tissues that highly scatter the light, e.g., skin, brain, sclera, blood, and vessel wall, whereas the second one consists of weakly scattering or nearly transparent tissues, such as cornea, eye lens, and thin histological sections of various types of biological tissues. Therefore, the optical properties of tissues in these groups are based, respectively, on multiple scattering (diffusion) approximation and single scattering. Although the approximation of photon diffusion is a cornerstone of optical imaging and near-infrared spectroscopy,2 it struggles to describe properly the propagation of polarized light in biological tissues both in single and multiple scattering regimes. In addition, neither single scattering nor multiple scattering approximations are not able to take into account the vector nature of the incident polarization and/or scattered light waves. The use of polarized light as an “instrumental probe” allows for assessing quantitatively optical anisotropy of the poly-crystalline structure of biological fluids and tissues.3–9 The polarization introscopy approach is well developed and known as Mueller-matrix (MM) microscopy in transmitted mode.10–15 In fact, MM microscopy is an example of a successful synthesis of instrumental imaging polarimetry, diverse theoretical modeling, and image processing, utilizing the regression model of optical anisotropy,11 logarithmic MM decomposition,12–15 Monte Carlo-based assessment of polarized light conversion,14 and statistical analysis of MM images and optical anisotropy maps.11,15 A considerable result of biological tissue screening, obtained with the MM microscopy, is highly promising for the clinical application and pre-clinical studies of the poly-crystalline structure of biological tissues.15 In particular, the possibility of obtaining quantitative optical metrics to characterize the evolution of gastric tissue from a healthy state through inflammation to cancer using MM microscopy of gastric biopsies, a regression model of optical anisotropy, and statistical analysis of the obtained images has been demonstrated.11 Extension of the applied functional capabilities of MM microscopy ensured the application of the differential MM formalism in the analysis of experimental data.12,13,15 On this basis, the maps of depolarization and polarization of fixed uncolored histological sections of human skin tissues were obtained. This allowed for mitigating the influence of tissue slice thickness variations and increasing the contrast of polarimetric images for tissue diagnosis. In addition, the use of MM microscopy data in combination with logarithmic decomposition and polarization Monte Carlo simulation (within the framework of Mie theory approximation) opens a way for qualitative and quantitative analysis of thin tissue sections to extract information about tissue microstructure,15,16 which is not available in conventional microscopy. It should be noted that the analyzed polarization introscopy methods3–6,8,9,17,18 and MM10–16 microscopy facilitate obtaining and visual analysis of the topological and coordinate structure of optical anisotropy maps of biological preparations. However, such analysis is somewhat subjective and does not provide a quantitative (objective) assessment of the severity or stages of pathology. Therefore, it is relevant to obtain a set of additional perceptible objective criteria (e.g., such as statistical moments of the first to fourth orders11,15) for MM characterization and differentiation of pathological conditions that are coordinate () and scale () localized in the biological tissue layer. However, the topological information about the optical anisotropy of the biological layer appears to be integrally averaged over all coordinates and geometric dimensions of MM map images within a quantitative statistical analysis. For statistical quantification of polarization-detected local variations in optical anisotropy parameters, statistical analysis of scale-selective samples from MM data derived from polarization-singular19–34 and scale-selective35–40 wavelet analysis may be most appropriate. The use of the polarization-singular approach defines the lines/surfaces at each point of a polarization-inhomogeneous field with indefinite (singular) parameters.19–22,41 These points are as follows:
The basic principles of complex vector singular analysis are formulated and described in details elsewhere,19,22–26 considered for optical fields,27–29 and practically implemented in biomedical imaging.30–34 Thus, for the first time, to our knowledge, biological tissues with a presence of linearly birefringence were characterized analytically in terms of the formation of linear and circular polarization singularities.27–29 A significant predominance of states in comparison with states is observed due to a more complex formation of circular polarization states and the domination of optically isotropic constituents within biological tissue morphology. The characteristic values of the fourth parameter of the Stokes vector were used as markers of polarization-singular states: for and for , whereas the distribution of polarization-singular states numbers (, ) was utilized to analyze images of biological tissue and fluid samples. It has been demonstrated that the third () and fourth () statistical moments characterizing the asymmetry and excess of the distributions and of singular points are sensitive to pathological changes in the poly-crystalline component of histological sections of biological tissues as well as in the poly-crystalline films of biological fluids. Such changes can lead to the necrosis of biological tissue morphological structure. As a result, the level of optical anisotropy decreases as well as the probability of for formation. Quantitatively, this leads to an increase in the value of statistical moments and . The wavelet analysis is one of the main analytical methods for scale-selective estimation of line pixel distributions of azimuth , ellipticity , and elements of MM .35,36 Utilizing the wavelet function, the distribution is expanded by the following equation: where is the basic function formed from the prototype function by offset and scaling , and the coefficients of this expansion are determined asThe wavelet transform allows for revealing both low-frequency and high-frequency characteristics of the distribution on the different coordinate scales (so-called “mathematical microscope”). Continuing the analogy with a mathematical microscope, the shift parameter fixes the focal point of the microscope, the scale factor shows the magnification, and the choice of the base wavelet is the optical properties of the microscope. In this study, the second derivative of the Gaussian function–MHAT wavelet is used. Such a function has a narrow energy spectrum and two moments equal to zero (zero and first) that suit well for the analysis of complex signals:37–40 The wavelet transforms of the one-dimensional distribution result in a two-dimensional array of amplitudes. The distribution of these values in space ( is the spatial scale, and is the spatial coordinate or localization) gives the information about the evolution of the relative contribution of components of different scales to the distribution under consideration and is called the spectrum of wavelet coefficients : The approbation of this approach demonstrates a significant improvement in the sensitivity and accuracy of MM polarimetry in the differential diagnosis of inflammatory and oncological conditions.30–36,42,43 However, the polarization-singular19–29,41,44,45 and scale-selective wavelet37–40,46,47 approaches in biomedical diagnosis require further developments. This study is aimed at identifying the analytical relationship between the polarization-singular states of the object field of optically thin (non-depolarizing) layers obtained from biological tissues and the characteristic values of their MM images registered in transmitted light. A robust method for expedited (up to 15 min) polarimetric-based differential diagnosis of local variations in the poly-crystalline structure of tissue samples containing various pathology abnormalities is presented. 2.Materials and Methods2.1.MM Imaging and Singular StatesDetermination of analytical relationships between the characteristic values of MM elements of the biological layer and polarization-singular states of its object field is based on using linear birefringence approximation for single light scattering by fibrillary networks of optically thin (attenuation coefficient ) layers of biological tissue. According to the birefringence model of spatially structured fibrillar networks,48–54 MM is presented by the following expression:17,18 whereHere, is the direction of the optical axis, determined by the orientation of the fibril position in the plane of the biological layer; defines the phase shift between linearly orthogonal polarized components of the laser beam amplitude; is the wavelength; characterises birefringence; and is the geometric layer thickness. Based on Eqs. (5) and (6), it is possible to determine the diagnostically important relationship between the characteristic values of MM elements and the formation conditions of “” and “” polarization-singular states formed by a birefringent fibrillar network (Table 1). Table 1Relationships between the characteristic values of the elements and azimuthal invariants of MM and polarization singularities.
In fact, most of the matrix elements presented in Table 1 are azimuthally dependent on the magnitude of the rotation of the sample plane relative to the irradiation direction.16–18 Therefore, for representative groups of biological tissue samples, it is necessary to use other azimuthally invariant MM functionals, which are also presented in Table 1. Using the information presented in Table 1, it is possible to determine a complete set of “”-points on the image of a biological object. The coordinate position of each point, in this way, corresponds to the conditions . Also, it is possible to determine a complete set of “”-points on the polarization image with arbitrary azimuths . Here, each point corresponds to the conditions . Finally, the “orthogonal” -points are determined. The formation of each -point is associated with the orthogonal orientations of the optical axes of birefringent fibrils as For the “azimuthal-invariant” polarization-singular states 2.2.Tissue SamplesTo validate the proposed method and show the possibility of determining the different types of tumors, the histological sections of prostate and uterine tissue were obtained using microtome with rapid freezing after radical prostatectomy. Four representative groups of obtained histological biopsy sections of tumors were formed: group 1:– adenoma samples; group 2: moderately differentiated ( on Gleason’s pattern scale) carcinoma samples; group 3: myoma samples; and group 4: uterine endometriosis samples. For the histological analysis, each tissue sample was first fixated with formalin (40% formaldehyde aqueous solution). After 24 h, the sample was washed with running water. Further, the sample was placed in alcohol with increasing concentrations (from 70% to 100%) to achieve tissue dehydration. After dehydration, the sample was fixated in a xylol-paraffin mixture for 1 to 2 h at a temperature of 52°C to 56°C. After that, the histological sections were cut using the standard microtome. Each section was further stained with hematoxylin-eosin. Further, the obtained sections were investigated by microscope and the position of the tumor was determined according to Gleason’s scale. Table 2 presents the optical and geometric parameters of the obtained histological sections of prostate tumor biopsies from both groups. Table 2Optical and geometric parameters of histological sections of prostate (groups 1 and 2) and uterine (groups 3 and 4) tissues.
The geometric thickness of histological sections of prostate and uterine tissues was determined by the standard values of scale of the freezing microtome. Variations in geometric thickness , within the plane of the histological sections () did not exceed and did not result in a significant change in optical thickness and single-scattering conditions. The measurement of the extinction coefficient of the prostate tissue samples was carried out according to the standard procedure of light attenuation measurement55 using an integral light scattering sphere.56 The sample preparation procedure was conducted in accordance with the principles of the Declaration of Helsinki and in compliance with the International Conference on Harmonization-Good Clinical Practice and local regulatory requirements. The study was reviewed and approved by the appropriate Independent Ethics Committees, and written informed consent was obtained from all subjects prior to the study initiation. 2.3.Experimental SetupThe experimental set up and the protocol of measurements of spatial distributions of the parameters of the Stokes vector and the elements of MM were developed earlier.30,31,48,49 Briefly, the optical setup is shown in Fig. 1. The setup utilized an He–Ne laser (Edmund Optics) emitting low-intensity () light at 633 nm. The light beam was further collimated and passed through the quarter-wave plate (Achromatic True Zero-Order Waveplate, APAW 15 mm, Astropribor, Ukraine) and polarizer (B+W XS-Pro Polarizer MRC Nano, Kaesemann, Germany). After that, the light beam was passed through the sample and projected to the CCD-camera (, DMK 41AU02.AS, The Imaging Source, Germany) using a polarization microobjective (CFI Achromat P, focal length: 30 mm, numerical aperture: 0.1, increase: , Nikon, Japan). Additional quarter-wave plates before the sample and after the microobjective were used for image analysis. Fig. 1Experimental setup. (1) He–Ne laser, (2) collimator, (3) stationary quarter-wave plate, (5), (8) mechanically movable quarter-wave plates, (4), (9) polarizer and analyzer, (6) histological section, (7) polarizing microobjective, (10) CCD camera. ![]() For the series of linear (0 deg, 90 deg, 45 deg) and right- circular polarized illuminating laser beams, the Stokes-vector parameter was defined for each pixel as Here, is the intensities of linearly (0 deg; 90 deg; 45 deg; 135 deg), right- () and left- () circularly polarized components of the filtered by means of polarizer 9 and quarter-wave plate 8 laser light. Finally, MM invariants were calculated as 2.4.Methods of MMI Processing2.4.1.Linear scanningTo obtain objective criteria for MM polarization-singular differentiation between optical properties of prostate adenoma, carcinoma, and myoma-endometriosis samples, the following procedure was performed. MM images of the element and a set of other MM invariants (see Table 1) were measured sequentially, and the coordinate grids of characteristic values were determined [Eq. (8)]. For example, matrix element and . By linear scanning along the pixel row of the photosensitive pad of digital camera 10 for each individual pixel (), the number () of characteristic values within the corresponding column , and so on, is determined. Further, sets of linear dependencies of points for MM image characteristic values were determined in two orthogonal directions as and Similarly for other MMIs, there were and , as well as and 2.4.2.Wavelet analysisThen, wavelet analysis of linear dependencies , was carried out, and two-dimensional arrays of wavelet coefficients and were determined. For the various scales of the MHAT function , sets of linear dependences of the wavelet coefficients amplitudes and were determined. For each scale , the central statistical moments of the first and second orders 48 were calculated, characterizing the average and dispersion of the distributions and . Further, the step of large-scale “macro” scanning () of MHAT function was selected. The difference between the values of each central statistical moments of the first and second orders was calculated . Then, the scale interval was determined, within the monotonic increase in the value stops. Also, within limits , a new series of values was calculated with a step of discrete scaled “micro” scan . Then, the optimal scale was determined following the condition . The mean , and standard deviations , were determined within the representative samplings of histological sections from group 1 to group 2 and group 3 to group 4. 2.4.3.Informational analysisTo differentiate pathological states of prostate and uterine, for each statistical moment , the sensitivity (), specificity (), and balanced accuracy () were calculated.57 Here, and are the numbers of correct and incorrect diagnoses, respectively, within group 2 and group 4; and and are the same within control group 1 and group 3. 3.Results and DiscussionThe results of the polarization-singular study (using MM images of invariants) of the polycrystalline structure for optically thin histological sections of benign (adenoma) and malignant (carcinoma) prostate tumor tissue samples are shown in Fig. 2. Here, Figs. 2(a) and 2(b) show the MMI of the adenoma [Fig. 2(a)] and carcinoma [Fig. 2(b)] samples. Figures 2(c)–2(f) show distributions of (Figs. 2(c) and 2(d)] and (Figs. 2(e) and 2(f)]. Similar dependencies of the number of characteristic values obtained for the orthogonal scanning direction () are presented [Figs. 2(g)–2(j)]. Fig. 2Spatial distributions of characteristic values of the MM images of histological sections of prostate adenoma (a) and carcinoma (b). Illustrative linear dependences of , , , and , respectively, for prostate adenoma (c), (e), (g), (i) and carcinoma (d), (f), (h), (j). See further details in the text. ![]() Comparative analysis of the obtained data (Fig. 2) revealed opposite tendencies in changes of distributions and during the formation of malignant carcinoma of the prostate regardless of scanning direction ( and ). The number of characteristic values decreases [Figs. 2(c) and 2(d) and 2(e) and 2(f)] and the number of characteristic values increases (Figs. 2(g) and 2(h) and 2(i) and 2(j)]. Physically, the obtained results can be explained by the fact that malignant necrotic changes in prostate tissue lead to degradation of its polycrystalline birefringent structure.17,18 As a consequence of this process, the “phase-shifting” ability of this layer decreases () and the probability of formation of C-polarization states decreases. By contrast, the probability of L-states formation increases, which corresponds to optically isotropic necrotically changed areas of carcinoma tissue. Quantitatively, these birefringence degradation processes of malignant prostate tumors are illustrated by the results of statistical () and informational () analysis of the , , and These are presented in Tables 3 and 4. Table 3Statistical informational parameters characterizing the distribution of f44(x,y), N((f44=±1),x), and N((f44=0),x) within both groups of prostate samples.
Table 4Statistical informational parameters characterizing the distribution of f44(x,y), N((f44=±1),y), and N((f44=0),y) within both groups of prostate samples.
The analysis of the presented data showed the low efficiency of the traditional MM polarimetry imaging method for discrimination between different types of prostate tumors (). At the same time, utilizing the statistical analysis of the distributions of characteristic values ( and ), the balanced accuracy of differential diagnosis is increased by 10% to 15% (). Similar results (within 5% of variation in the value of the balanced accuracy ) were obtained using statistical and polarization-singular analysis of the set of other MM invariants (Table 5). Table 5Operational characteristics of MM invariants and polarization-singularity methods diagnostic power.
This result can be related to the fact that all MM images that characterize optical anisotropy of the polycrystalline structure for histological sections of prostate tumor tissue samples are functionalities of a single physical mechanism—phase-shifting capacity of linear birefringence—. In addition, for both scanning directions ( and ), the statistical () and informational () parameters of both methods are close enough—the differences between lie within 8% to 15%, and variations of diagnostic accuracy do not exceed 2% to 3%. In addition, the obtained result can be explained by the fact that pathological changes of fibrillar networks of prostate tumor samples are sufficiently azimuthally symmetric. On the other hand, for other tissue types and pathologies, other scenarios of birefringent polycrystalline structure changes can also be realized. For this purpose, we performed an additional set of studies aimed at the differential diagnosis of extragenital endometriosis (group 3 and group 4) by methods of azimuthal-invariant MM polarimetry and polarization-singular MM image analysis (see Fig. 3, Tables 6Table 7–8). Fig. 3Spatial distributions of characteristic values of the MM images of histological sections of prostate adenoma (a) and carcinoma (b). Illustrative linear dependences of , , , and , respectively, for prostate adenoma (c), (e), (g), (i) and carcinoma (d), (f), (h), (j). See further details in the text. ![]() Table 6Statistical informational parameters characterizing the distribution of f44(x,y), N((f44=±1),x), and N((f44=0),x) within both groups of uterine samples.
Table 7Statistical informational parameters characterizing the distribution of f44(x,y), N((f44=±1),y), and N((f44=0),y) within both groups of uterine samples.
Table 8Operational characteristics of MM invariants (MMI) and polarization-singularity methods diagnostic power.
Comparative analysis of the obtained data (Fig. 3) revealed different (opposite to Fig. 2) tendencies in and distributions changing during uterine endometriosis formation in both scanning directions ( and ): the number of characteristic values increases [Figs. 3(c) and 3(d) and 3(g) and 3(h)], whereas the number of characteristic values decreases [Figs. 3(e) and 3(f) and 3(i) and 3(j)]. The obtained results can be explained by the fact that pathological endometriosis overgrowth of fibrillar networks of connective tissue leads to an increase in the level of linear birefringence.31,32,48,49 As a consequence of this process, the “phase-shifting” ability of this layer increases , and the probability of the formation of C-polarization states increases as well. By contrast, the probability of forming L states, which correspond to optically isotropic altered regions of the uterine endometrium, decreases. Analysis of the data presented in Tables 6Table 7–8 revealed a slightly higher () diagnostic efficiency of myoma and extragenital endometriosis differentiation by azimuthal-invariant MM polarimetry: . Polarization-singular processing of the data obtained provides a further increase in the level of balanced accuracy to the level of . Similar results (within 5% variation in the value of the balanced accuracy ) were obtained using statistical and polarization-singular analysis of the set of other MM invariants (Table 8). At that, for both scanning directions ( and ), the differences between M,D increased 12% to 20%, and the variation in the accuracy was 7% to 9%. The obtained results can be explained by the fact that the pathological formation of newly formed fibrillar networks of endometrial connective tissue leads to an increase in structural anisotropy48,49 (linear birefringence), the polarization manifestations of which may be azimuthally asymmetric. The main factor limiting the accuracy of MM differential diagnosis of pathological changes is the integral averaging of experimentally obtained information about optical anisotropy over all coordinates and geometric dimensions of the morphological structure of biological tissues.10–18 We show (Tables 3–8) that the use of polarization-singular samples from the whole array of MM invariant values provides an increase in the accuracy of differential diagnosis of pathological conditions of the prostate and uterine endometrium tissues by 15% to 20%. It should be noted that the mentioned processes are scale-dependent. Oncological changes that are accompanied by necrotic destruction of morphological structure are localized in large-scale areas of birefringent fibrillary networks of the prostate. By contrast, the formation (growth) of fibrillar networks of connective tissue of the uterine endometrium are localized in small-scale areas. Based on this, we next investigated additional possibilities of scale-selective wavelet analysis [Eqs. (1)–(4)] of distributions of number of characteristic MM invariant values to improve the accuracy of differential diagnostics of biological tissues from different human organs. Figures 4 and 5 show the wavelet transform maps [Figs. 4, 5(a), and 5(b)] of distributions , , the linear dependencies of the amplitudes of the wavelet coefficients [Figs. 4, 5(c), and 5(d)] and [Figs. 4(e) and 4(f)] on the optimal scale of the MHAT function of MM image characteristic values for adenoma and carcinoma (Fig. 4) and myoma and endometriosis (Fig. 5) tissues. Fig. 4Wavelet transform of distributions and for (a) adenoma and (b) carcinoma tissues. Linear dependencies of the amplitudes of the wavelet coefficients and on the optimal scale of the MHAT function of MM image characteristic values for (c), (e) adenoma and (d), (f) carcinoma tissues, respectively. ![]() Fig. 5Wavelet transform of distributions and for (a) adenoma and (b) carcinoma tissues. Linear dependencies of the amplitudes of the wavelet coefficients and on the optimal scale of the MHAT function of MM image characteristic values for (c), (e) myoma and (d), (f) endometriosis tissues, respectively. ![]() A comparative analysis of and distributions revealed opposite tendencies in the formation of the magnitude and range of variation of their amplitudes . First, for the carcinoma tissues, the range of amplitude changes of wavelet coefficients for was smaller than that of the adenoma tissues. At the same time, the range of amplitude changes of wavelet coefficients for was higher for adenoma tissues than for the carcinoma. For the endometrium tissues, the range of amplitude changes of wavelet coefficients for was higher compared with the myoma tissues. At the same time, the amplitude range of changes of wavelet coefficients for was smaller for endometrium tissues than for myoma. From a physical point of view, the obtained results can be explained by the fact that cancer development leads to the destruction of birefringent large-scale localized domains of the prostate tissue. Thus, in corresponding areas, the value of the phase shifts decreases. In this way, the probability of C -states formation and the number of characteristic values of MM image are reduced. Therefore, for a given scale , during scanning , the maximum extrema of the distributions of the wavelet coefficients are formed. The opposite picture takes place in the wavelet analysis of distributions characterizing the number of L-states corresponding to necrotically changed (almost optically isotropic) areas of adenocarcinoma tissues, which are characterized by values . As a result, for adenocarcinoma samples, the average and dispersion characterized the distributions of wavelet coefficients amplitudes of the number of L-states. The opposite picture takes place for the statistical parameters characterizing the distributions . For the pathological growth of fibrillar networks of endometrium connective tissue, the opposite tendencies to that of prostate tissue are realized. Specifically, for myoma samples, the average and dispersion, characterizing the distributions of the amplitudes of the wavelet coefficients of the number of L-states, compared more to the same parameters of the carcinoma samples. The opposite picture takes place for the statistical parameters of prostate tumors samples. The quantitative data for the distributions and for prostate and uterine tissues are presented in Tables 9 and 10, respectively. Table 9Statistical and informational parameters characterizing the distributions QA(L,b) and QA(C,b) within both groups of prostate samples.
Table 10Statistical and informational parameters characterizing the distributions QA(L,b) and QA(C,b) within both groups of uterine samples.
The accuracy of the wavelet analysis of the distributions of MM image characteristic values in the differentiation of the tumor states for the prostate tissue reaches an excellent quality of , and for uterine tissue, . Thus, the proposed method of wavelet analysis of the characteristic value distributions for MM images of linear birefringence significantly expands the functionality of the traditional polarization mapping of histological sections with minor changes in the optical anisotropy of fibrillar networks. 4.ConclusionsIn this study, an analytical relationship between the characteristic values of individual matrix elements and polarization singularities of microscopic images of birefringent fibrillar networks of biological tissues were established within the framework of an MM model of phase anisotropy. The elements of MM treated with the wavelet analysis were used to diagnose the local manifestations of localized changes in the magnitude of birefringence of the polycrystalline fibrillar compounds within biological tissue. The statistical analysis of characteristic values of spatial distributions of the obtained MM images demonstrates a high potential for differentiating the benign and malignant states of the prostate and uterine tissues with excellent accuracy. AcknowledgmentsThe authors acknowledge the support of the National Research Foundation of Ukraine, Project 2020.02/0061; ATTRACT II META-HiLight project funded by the European Union’s Horizon 2020 research and innovative programme under Grant Agreement No. 101004462, the Academy of Finland (Grant Projects 314639, 325097, 351068), the Leverhulme Trust, and The Royal Society (Ref. No.: APX111232 APEX Awards 2021). ReferencesV. V. Tuchin,
“Light scattering study of tissues,”
Physics-Uspekhi, 40
(5), 495 https://doi.org/10.1070/PU1997v040n05ABEH000236 PHUSEY 1063-7869
(1997).
Google Scholar
T. Durduran et al.,
“Diffuse optics for tissue monitoring and tomography,”
Rep. Prog. Phys., 73
(7), 076701 https://doi.org/10.1088/0034-4885/73/7/076701 RPPHAG 0034-4885
(2010).
Google Scholar
A. Ushenko et al.,
“Stokes-correlometry analysis of biological tissues with polycrystalline structure,”
IEEE J. Sel. Top. Quantum Electron., 25
(1), 7101612 https://doi.org/10.1109/JSTQE.2018.2865443 IJSQEN 1077-260X
(2019).
Google Scholar
V. Ushenko et al.,
“3D Mueller-matrix diffusive tomography of polycrystalline blood films for cancer diagnosis,”
Photonics, 5
(4), 54 https://doi.org/10.3390/photonics5040054
(2018).
Google Scholar
V. A. Ushenko et al.,
“Biomedical application of jones-matrix tomography to polycrystalline films of biological fluids,”
J. Innov. Opt. Health Sci., 12
(6), 1950017 https://doi.org/10.1142/S1793545819500172
(2019).
Google Scholar
M. Borovkova et al.,
“Mueller-matrix-based polarization imaging and quantitative assessment of optically anisotropic polycrystalline networks,”
PLOS One, 14
(5), e0214494 https://doi.org/10.1371/journal.pone.0214494 POLNCL 1932-6203
(2019).
Google Scholar
M. A. Borovkova et al.,
“Role of scattering and birefringence in phase retardation revealed by locus of stokes vector on poincare sphere,”
J. Biomed. Opt., 25
(5), 057001 https://doi.org/10.1117/1.JBO.25.5.057001 JBOPFO 1083-3668
(2020).
Google Scholar
M. Peyvasteh et al.,
“3D Mueller-matrix azimuthally-invariant tomography of polycrystalline structure of benign and malignant tumors,”
Laser Phys. Lett., 17
(11), 115606 https://doi.org/10.1088/1612-202X/abbee0 1612-2011
(2020).
Google Scholar
O. Sieryi et al.,
“Optical anisotropy composition of benign and malignant prostate tissues revealed by Mueller matrix imaging,”
Biomed. Opt. Express., 13
(11), 6019
–6034 https://doi.org/10.1364/BOE.464420 BOEICL 2156-7085
(2022).
Google Scholar
H. R. Lee et al.,
“Digital histology of tissue with Mueller microscopy and fastDBSCAN,”
Appl. Opt., 61
(32), 9616
–9624 https://doi.org/10.1364/AO.473095 APOPAI 0003-6935
(2022).
Google Scholar
M. Kim et al.,
“Optical diagnosis of gastric tissue biopsies with mueller microscopy and statistical analysis,”
J. Eur. Opt. Soc., 18
(2), 10 https://doi.org/10.1051/jeos/2022011 1990-2573
(2022).
Google Scholar
H. R. Lee et al.,
“Digital histology with Mueller microscopy: how to mitigate an impact of tissue cut thickness fluctuations,”
J. Biomed. Opt., 24
(7),
–076004 https://doi.org/10.1117/1.JBO.24.7.076004 JBOPFO 1083-3668
(2019).
Google Scholar
P. Li et al.,
“Analysis of tissue microstructure with mueller microscopy: logarithmic decomposition and Monte Carlo modeling,”
J. Biomed. Opt., 25
(1),
–015002 https://doi.org/10.1117/1.JBO.25.1.015002 JBOPFO 1083-3668
(2020).
Google Scholar
H. R. Lee et al.,
“Mueller microscopy of anisotropic scattering media: theory and experiments,”
Proc. SPIE, 10677 1067718 https://doi.org/10.1117/12.2306943 PSISDG 0277-786X
(2018).
Google Scholar
H. Ma, H. He, J. C. Ramella-Roman,
“Mueller matrix microscopy,”
Polarized Light in Biomedical Imaging and Sensing: Clinical and Preclinical Applications, 281
–320 Springer(
(2022). Google Scholar
I. Meglinski et al.,
“Scale-selective and spatial-frequency correlometry of polarization-inhomogeneous field,”
Shedding the Polarized Light on Biological Tissues, 33
–59 Springer, Singapore
(2021). Google Scholar
N. Ghosh and I. Vitkin,
“Tissue polarimetry: concepts, challenges, applications, and outlook,”
J. Biomed. Opt., 16
(11), 110801 https://doi.org/10.1117/1.3652896 JBOPFO 1083-3668
(2011).
Google Scholar
S. Jacques,
“Polarized light imaging of biological tissues,”
Handbook of Biomedical Optics, 649
–669 CRC Press, Boca Raton, Florida
(2011). Google Scholar
M. Soskin, V. Denisenko and I. Freund,
“Optical polarization singularities and elliptic stationary points,”
Opt. Lett., 28
(16), 1475
–1477 https://doi.org/10.1364/OL.28.001475 OPLEDP 0146-9592
(2003).
Google Scholar
M. Dennis,
“Polarization singularities in paraxial vector fields: morphology and statistics,”
Opt. Commun., 213
(4-6), 201
–221 https://doi.org/10.1016/S0030-4018(02)02088-6 OPCOB8 0030-4018
(2002).
Google Scholar
J. V. Hajnal and J. F. Nye,
“Singularities in the transverse fields of electromagnetic waves. I. Theory,”
Proc. R. Soc. A Math. Phys. Sci., 414
(1847), 433
–446 https://doi.org/10.1098/rspa.1987.0153
(1987).
Google Scholar
J. V. Hajnal and J. F. Nye,
“Singularities in the transverse fields of electromagnetic waves. II. Observations on the electric field,”
Proc. R. Soc. A Math. Phys. Sci., 414
(1847), 447
–468 https://doi.org/10.1098/rspa.1987.0154
(1987).
Google Scholar
J. F. Nye,
“Lines of circular polarization in electromagnetic wave fields,”
Proc. R. Soc. A Math. Phys. Sci., 389
(1797), 279
–290 https://doi.org/10.1098/rspa.1983.0109
(1983).
Google Scholar
K. Bliokh,
“Geometrical optics of beams with vortices: berry phase and orbital angular momentum hall effect,”
Phys. Rev. Lett., 97
(4), 043901 https://doi.org/10.1103/PhysRevLett.97.043901 PRLTAO 0031-9007
(2006).
Google Scholar
F. Flossmann et al.,
“Polarization singularities from unfolding an optical vortex through a birefringent crystal,”
Phys. Rev. Lett., 95
(25), 253901 https://doi.org/10.1103/PhysRevLett.95.253901 PRLTAO 0031-9007
(2005).
Google Scholar
F. Flossmann et al.,
“Stokes parameters in the unfolding of an optical vortex through a birefringent crystal,”
Opt. Exp., 14
(23), 11402
–11411 https://doi.org/10.1364/OE.14.011402 OPEXFF 1094-4087
(2006).
Google Scholar
O. Angelsky et al.,
“Singularities in vectoral fields,”
Proc. SPIE, 3904 40
–54 https://doi.org/10.1117/12.370443 PSISDG 0277-786X
(1999).
Google Scholar
I. Freund et al.,
“Stokes singularity relations,”
Opt. Lett., 27
(7), 545
–547 https://doi.org/10.1364/OL.27.000545 OPLEDP 0146-9592
(2002).
Google Scholar
O. Angelsky et al.,
“The relationship between topological characteristics of component vortices and polarization singularities,”
Opt. Commun., 207
(1–6), 57
–65 https://doi.org/10.1016/S0030-4018(02)01479-7 OPCOB8 0030-4018
(2002).
Google Scholar
O. Angelsky et al.,
“Polarization singularities of the object field of skin surface,”
J. Phys. D. Appl. Phys., 39
(16), 3547
–3558 https://doi.org/10.1088/0022-3727/39/16/005 JPAPBE 0022-3727
(2006).
Google Scholar
O. Angelsky et al.,
“Correlation- and singular-optical approaches in diagnostics of polarization inhomogeneity of coherent optical fields from biological tissues,”
Ukr. J. Phys. Opt., 8
(2), 106
–123 https://doi.org/10.3116/16091833/8/2/106/2007
(2007).
Google Scholar
Y. Ushenko, A. Angelskaya and D. Burkovets,
“On the interconnection between correlation and singular-optics approaches in polarization diagnostics of fields from biological tissues,”
Proc. SPIE, 7008 700821 https://doi.org/10.1117/12.797364 PSISDG 0277-786X
(2008).
Google Scholar
Y. Ushenko and O. Telenga,
“Polarization-singular processing of biological layers laser images to diagnose and classify their optical properties,”
Proc. SPIE, 8338 83380U https://doi.org/10.1117/12.920074 PSISDG 0277-786X
(2011).
Google Scholar
Y. Ushenko et al.,
“Statistical, fractal, and singular processing of phase images of hominal blood plasma during the diagnostics of breast cancer,”
J. Flow Vis. Image Process., 18
(3), 185
–197 https://doi.org/10.1615/JFlowVisImageProc.2011003254 JFVPEI 1065-3090
(2011).
Google Scholar
Y. O. Ushenko et al.,
“Wavelet analysis for Mueller matrix images of biological crystal networks,”
Semicond. Phys. Quantum Electron., 12 391
–398 https://doi.org/10.15407/spqeo12.04.391 1560-8034
(2009).
Google Scholar
Y. Ushenko et al.,
“Wavelet-analysis for laser images of blood plasma,”
Adv. Electr. Comput. Eng., 11
(2), 55
–62 https://doi.org/10.4316/aece.2011.02009
(2011).
Google Scholar
I. Daubechies, Ten Lectures on Wavelets, Society for Industrial and Applied Mathematics(
(1992). Google Scholar
A. Cohen, I. Daubechies and P. Vial,
“Wavelets on the interval and fast wavelet transforms,”
Appl. Comput. Harmon. Anal., 1
(1), 54
–81 https://doi.org/10.1006/acha.1993.1005 ACOHE9 1063-5203
(1993).
Google Scholar
Y. Meyer,
“Wavelets: Algorithms and Applications,”
Society for Industrial and Applied Mathematics (SIAM), Philadelphia
(1993). Google Scholar
S. Mallat,
“A theory for multiresolution signal decomposition: the wavelet representation,”
IEEE Trans. Pattern Anal. Mach. Intell., 11
(7), 674
–693 https://doi.org/10.1109/34.192463 ITPIDJ 0162-8828
(1989).
Google Scholar
I. Freund, M. Soskin and A. Mokhun,
“Elliptic critical points in paraxial optical fields,”
Opt. Commun., 208
(4-6), 223
–253 https://doi.org/10.1016/S0030-4018(02)01585-7 OPCOB8 0030-4018
(2002).
Google Scholar
V. Bachinsky et al.,
“Wavelet analysis for polarization maps of networks formed by liquid biological crystals in blood plasma: statistical and fractal approaches,”
Semicond. Phys. Quantum Electron., 13 189
–201 https://doi.org/10.15407/spqeo13.02.189 1560-8034
(2010).
Google Scholar
Y. Ushenko,
“Wavelet analysis of polarization maps of polycrystalline biological fluids networks,”
Opto-Electron. Rev., 19
(4), 425
–434 https://doi.org/10.2478/s11772-011-0041-3 OELREM 1230-3402
(2011).
Google Scholar
J. Nye,
“Line singularities in wave fields,”
Philos. Trans. R. Soc. A, 355
(1731), 2065
–2069 https://doi.org/10.1098/rsta.1997.0107 PTRMAD 1364-503X
(1997).
Google Scholar
M. Berry and M. Dennis,
“Polarization singularities in isotropic random vector waves,”
Proc. Math. Phys. Eng. Sci., 457
(2005), 141
–155 https://doi.org/10.1098/rspa.2000.0660
(2001).
Google Scholar
I. Daubechies,
“Wavelets on the interval,”
Progress in Wavelet Analysis and Applications: Proceedings of the International Conference” Wavelets and Applications,” Toulouse, France-June 1992, 95
–107 Atlantica Séguier Frontières(
(1993). Google Scholar
M. Farge,
“Wavelet transforms and their applications to turbulence,”
Annu. Rev. Fluid Mech., 24
(1), 395
–458 https://doi.org/10.1146/annurev.fl.24.010192.002143 ARVFA3 0066-4189
(1992).
Google Scholar
O. V. Angelsky et al.,
“Statistical, correlation, and topological approaches in diagnostics of the structure and physiological state of birefringent biological tissues,”
Handbook of Photonics for Biomedical Science, 283
–322 CRC Press, Taylor and Francis Publishing, London
(2010). Google Scholar
Y. A. Ushenko et al.,
“Diagnostics of structure and physiological state of birefringent biological tissues: Statistical, correlation and topological approaches,”
Handbook of Coherent-Domain Optical Methods: Biomedical Diagnostics, Environmental Monitoring, and Materials Science, 107
–148 Springer New York, New York
(2013). Google Scholar
R. Chipman,
“Polarimetry,”
Handbook of Optics: Volume I-Geometrical and Physical Optics, Polarized Light, Components and Instruments, 22.1
–22.37 McGraw-Hill Education(
(2010). Google Scholar
M. K. Swami, H. S. Patel and P. K. Gupta,
“Conversion of 3 × 3 Mueller matrix to 4 × 4 Mueller matrix for non-depolarizing samples,”
Opt. Commun., 286 18
–22 https://doi.org/10.1016/j.optcom.2012.08.094 OPCOB8 0030-4018
(2013).
Google Scholar
N. Ghosh, M. Wood, A. Vitkin,
“Polarized light assessment of complex turbid media such as biological tissues using mueller matrix decomposition,”
Handbook of Photonics for Biomedical Science, 253
–282 CRC Press, Taylor and Francis Publishing, London
(2010). Google Scholar
D. Layden, N. Ghosh, A. Vitkin,
“Quantitative polarimetry for tissue characterization and diagnosis,”
Advanced Biophotonics: Tissue Optical Sectioning, 73
–108 CRC Press, Boca Raton, Florida
(2013). Google Scholar
A. Vitkin, N. Ghosh, A. D. Martino,
“Tissue polarimetry,”
Photonics: Scientific Foundations, Technology and Application, IV 239
–321 John Wiley & Sons, Ltd., Hoboken, New Jersey
(2015). Google Scholar
R. Marchesini et al.,
“Extinction and absorption coefficients and scattering phase functions of human tissues in vitro,”
Appl. Opt., 28
(12), 2318
–2324 https://doi.org/10.1364/AO.28.002318 APOPAI 0003-6935
(1989).
Google Scholar
D. K. Edwards et al.,
“Integrating sphere for imperfectly diffuse samples,”
J. Opt. Soc. Am., 51
(11), 1279
–1288 https://doi.org/10.1364/JOSA.51.001279 JOSAAH 0030-3941
(1961).
Google Scholar
S. P. Robinson, Principles of Forensic Medicine, Cambridge University Press(
(1996). Google Scholar
BiographyAnton Sdobnov has an MSc in physics (2013) and a PhD in 2017 from Saratov State University, Russia, and a DSc (Tech) in applied electronics (2022) from the University of Oulu, Finland. He was a visiting scholar at the University Hospital Charite, Berlin, Germany in 2014–2015, and the Weizmann Institute of Science, Rehovot, Israel in 2018 and 2019, and recipient of Finish CIMO Fellowship grant (2016), UDUFI Fellowship grant (2017) and Finnish Cultural Foundation Grant (2018). He is author and co-author of more than 30 papers in peer-review scientific journals and proceedings of international conferences. His current research interests include dynamic light scattering, laser speckle contrast imaging, confocal Raman microscopy, optical clearing, tissue polarimetry, orbital angular momentum of light and biomedical optics. Volodymir A. Ushenko is an associate professor at the Yuriy Fedkovych Chernivtsi National University. He received his BS and MS degrees in optics from the Yuriy Fedkovych Chernivtsi National University in 2008 and 2010, respectively, and his PhD in optics, laser physics from the Kyiv National University in 2015. He is author and co-author of more than 50 peer-reviewed scientific journal papers. His current research interests include laser polarimetry, holography, cancer detection, and tissue diagnosis. Liliya Trifonyuk, PhD in medicine (2020), is a clinical doctor-surgeon-urologist at the Urology Department of Rivne Regional Clinical Hospital and Rivne Regional Oncology Dispensary. She has been borrowing the most modern European experience in the treatment of urological patients for the third year in one of the largest and best clinics in Poland – the Military Medical Institute in Warsaw. She is president of the All-Ukrainian Association of Reconstructive Urogynecology, the leading expert of the Department of Health of the Rivne Regional State Administration in the specialty “Urology,” and member of the European Association of Urologists. Oksana Bakun, PhD, doctorate, candidate of medical sciences, graduated from the Bukovinian State Medical Academy in 2002. She completed an internship on the basis of Maternity Home No1 at the Bukovinian State Medical Academy with the specialty obstetrics and gynecology in 2004, and studied at the graduate school of the Bukovinian State Medical University 2004–2007. Presently, she is medical doctor at the Obstetrics and Gynecology Department, Bukovinian State Medical University, Chernivtsi, Ukraine. Marta Garazdyuk graduated with honours from the Bukovyna State Medical University in 2010 with the recommendation for the advanced scientific studies; completed an internship in forensic medical examination at the Kyiv Medical Academy of Postgraduate Education named after P.L. Shupyk in 2012, and PhD in 2018 with the subject “Temporal monitoring of postmortem changes in autofluorescence of cerebrospinal fluid films in determining the time of death.” She is an associate professor at the Department of Forensic Medicine and Medical Law at the Bukovinian State Medical University. Author and co-author of more than 70 scientific papers, including 12 utility model patents. Irina V. Soltys received BSc and MSc degrees in optics in 2009, 2010, respectively, and a PhD in optics, laser physics from the Yuriy Fedkovych Chernivtsi National University in 2013. She is an associate professor at the Chernivtsi National University, author and co-author of more than 60 papers in peer-reviewed scientific journals and five book chapters. Her current research interests include laser polarimetry, holography, cancer detection, and biomedical tissue diagnosis. Olexander Dubolazov has BSc and MSc degrees in optics in 2006 and 2007, respectively, and a PhD in optics, laser physics from the Yuriy Fedkovych Chernivtsi National University in 2010. He is currently professor at the Yuriy Fedkovych Chernivtsi National University. He is deputy director in research at the Department of Optics and a head of division of the Academy of Engineering Sciences of Ukraine in the Chernivtsi National University. He is author and co-author of more than 100 papers in peer-reviewed scientific journals and five book chapters. His current research interests include laser polarimetry, holography, cancer detection, and biomedical tissue diagnosis. Olexander G. Ushenko has BSc and MSc degrees in optics from the Yuriy Fedkovych Chernivtsi State University in 1975 and 1977, respectively, and PhD and DSc in degrees optics and laser physics, respectively, in 1983 and 2000 from the Yuriy Fedkovych Chernivtsi State University. He is professor and head of Optics and Publishing Department at the Yuriy Fedkovych Chernivtsi National University. He is member of the Academy of Engineering Sciences of Ukraine and Academician of the Academy of Sciences of the Higher School of Ukraine. He is author and co-author of more than 200 papers in peer-reviewed scientific journals, 60 patents and 20 monographies. His current research interests include laser polarimetry, auto-fluorescence polarimetry, statistical, fractal, and correlation imaging analysis, singular optics, polarization interferometry, and digital holography. Yuriy A. Ushenko received an MSc in telecommunications in 2003, a PhD in optics and laser physics in 2006, and a DSc in optics and laser physics from Taras Shevchenko National University of Kyiv in 2015. Presently he is professor and head of the Computer Science Department at the Yuriy Fedkovych Chernivtsi National University, Ukraine. He is director of Cluster Bit of the regional Bukovinian IT Cluster. He is author and co-author of more than 150 papers in peer-reviewed scientific journals and seven monographs. His research interests include AI and data analysis, computer vision and pattern recognition, 3D laser polarimetry, optics and photonics, and biomedical optics. Alexander Bykov has an MSc in physics (2005), a PhD in laser physics and nonlinear optics (2008) from Moscow State University, Russia, and a DSc (Tech) in applied electronics (2010) from the University of Oulu, Finland. He is currently an associate professor and the Biophotonics Group Leader at the Opto-Electronics and Measurement Techniques (OPEM) Research Unit in the University of Oulu. He is a visiting researcher in Beckman Laser Institute, University of California at Irvine, US and Ecole Polytechnique, Palaiseau, France. He established a long-lasting collaboration between OPEM and Northern Finland Biobank Borealis. He is author and co-author of over 120 research papers published in peer-reviewed scientific journals and proceedings of international conferences, and 4 book chapters; over 50 presentations at the international conferences, symposia and workshops, including 30+ invited lectures. His research interests are in the area of polarization and hyperspectral imaging/sensing, optical properties of biotissues, numerical simulations, as well as signal and image processing. Igor Meglinski, PhD 1997, studies at the interface between Saratov State University, Russia, and University of Pennsylvania, United States. He is professor of quantum biophotonics and biomedical engineering at Aston University (UK). He is author of over 400 publications in peer-reviewed scientific journals, proceedings of conferences, 20+ book chapters, 7 books, and several patents. He has made over 750 presentations at the major international conferences, symposia, and workshops, including over 250 keynotes, plenary, and invited lectures at the major international conferences in the field and over 100 invited lectures at the universities and international research centers. He is Chartered Physicist (CPhys), Chartered Engineer (CEng), Fellow of the Institute of Physics, Fellow of Royal Microscopical Society (FRMS), Fellow of SPIE and Fellow of Optica (formerly OSA). His current research interest lies with advanced biomedical imaging, polarization of light, shaped light with orbital angular momentum, light-tissue interaction, dynamic light scattering and waves propagation in random tissue-like scattering medium. |
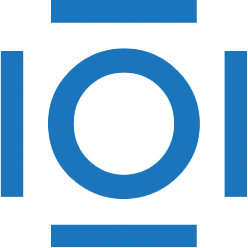
CITATIONS
Cited by 6 scholarly publications.
Tissues
Wavelets
Biomedical optics
Polarimetry
Biological samples
Prostate
Tissue optics