|
ACCESS THE FULL ARTICLE
No SPIE Account? Create one
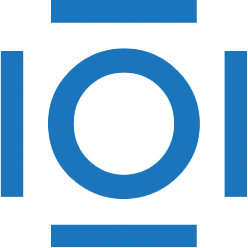
CITATIONS
Cited by 6 scholarly publications.
Edge detection
Sensors
Image segmentation
Detection and tracking algorithms
Single mode fibers
Fuzzy logic
Signal to noise ratio