Visual target tracking is an important function in real-time video monitoring application, whose performance determines the implementation of many advanced tasks. At present, Siamese-network trackers based on template matching show great potential. It has the advantage of balance between accuracy and speed, due to the pre-trained convolutional network to extract deep features for target representation and off-line tracking of each frame. During tracking, however, the target template feature is only obtained from the first frame of the video in the existing algorithms. The tracking performance is completely depending on the framework of template matching, resulting in the independence of frames and ignoring the feature of inter-frame connection of video sequence. Therefore, the existing algorithms do not perform well in the face of large deformation and severe occlusion. We propose a long short-term memory (LSTM) improved Siamese network (LSiam) model, which takes advantages of both time-domain regression capability of the LSTM and the balanced ability in tracking accuracy and speed of Siamese network. It focus on the temporal and spatial correlation information between video sequences to improve the traditional Siamese-network trackers with an LSTM prediction module. In addition, an improved template updating module is constructed to combine the original template with the changed appearance. The proposed model is verified in two types of difficult scenarios: deformation challenge and occlusion challenge. Experimental results show that our proposed approach can get better performance in terms of tracking accuracy. |
ACCESS THE FULL ARTICLE
No SPIE Account? Create one
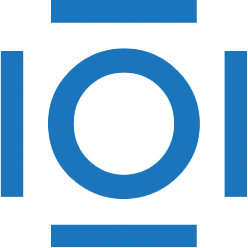
CITATIONS
Cited by 2 scholarly publications.
Detection and tracking algorithms
Video
Optical tracking
Lithium
Neural networks
Data modeling
Convolution