The skeleton-based action recognition task has recently drawn much attention in computer vision, and graph convolutional networks (GCNs) have shown great advantages in this task. However, in shallow layers of GCNs, the sharing of convolution kernels in regular graph convolution (RGC) may result in the underutilization of some convolution kernels due to the particularity of the skeleton data. To capture richer locally discriminative action features, we propose the exclusive kernel graph convolution (EKGC) for the shallow layers of GCNs, which assigns the vertexes exclusive convolution kernels for graph convolution. At the same time, following the mixed graph convolution strategy, RGC is retained in the deep layers of the network, and the mixed graph convolutional network (MGCN) is proposed to utilize EKGC and RGC reasonably in all layers of the network. In addition, as a new form of skeleton information in GCN-based methods, angle information is explored in our work. Compared with joint data and bone data, angle data have better invariance against rotation and scaling, and they expand the relationships between skeletons and enrich the data representation of action. Finally, our final model, the multi-stream MGCN, is validated on two challenging datasets, achieving the state-of-the-art performance on the NTU-RGBD dataset and making competitive performance on a kinetics-skeleton dataset. |
ACCESS THE FULL ARTICLE
No SPIE Account? Create one
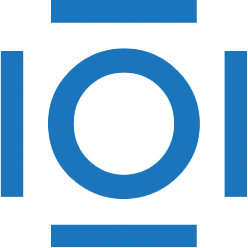
CITATIONS
Cited by 2 scholarly publications.
Convolution
Bone
Data modeling
Performance modeling
Motion models
Spine
3D modeling