Visible-infrared (IR) person re-identification is a technology that matches the identity of the same person in two modalities. The main challenge is to discover the differentiations between different identities and the similarities between the two modalities. To solve this problem, we propose a cross-modality consistency learning network, which jointly considers cross-modal learning and distillation learning. It consists of two associated components: the feature adaptation network (FANet) and the modality learning module (MLM). The FANet combines global and local information to extract more discriminative features on the same identity images, and MLM is used to alleviate modal differences between visible and IR images. Our model could adaptively select the high-quality person image according to the potential contribution of each image, to avoid negative knowledge transfer. Extensive experiments on the public SYSU-MM01 and RegDB datasets demonstrate the superiority of our approach over the current state-of-the-art technologies. |
ACCESS THE FULL ARTICLE
No SPIE Account? Create one
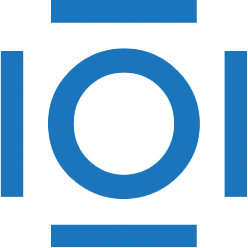
CITATIONS
Cited by 1 scholarly publication.
RGB color model
Infrared imaging
Education and training
Feature extraction
Visible radiation
Data modeling
Cameras