We present a skin lesion diagnosis system that segments the lesion and classifies it as melanoma or nonmelanoma. The proposed system is capable to deal with skin lesion images acquired by standard consumer-grade cameras and dermascopes. In order to suppress the image artifacts and enhance the lesion area, we propose an illumination correction strategy which consists of filtering in frequency and spatial domains. We introduce a hybrid model for lesion segmentation, which forms texture segments of the illumination corrected image using a factorization technique. Then based on the texture distinctiveness of the corrected and the texture segmented images, the saliency maps are computed, which are combined to decide lesion texture segments. In order to classify the segmented lesion, we propose a multimodal feature set composed of texture-, shape-, and color-based features. Classification performance of the multimodal features is evaluated using support vector machine, decision trees, and Mahalanobis distance classifiers. We evaluate the performance of the proposed system qualitatively and quantitatively. For the consumer-grade camera skin images dataset and ISIC 2017 dermascopic images dataset, the average segmentation accuracies are 98.4% and 95.4%, respectively; the classification accuracies are 98.06% and 93.95%, respectively. |
ACCESS THE FULL ARTICLE
No SPIE Account? Create one
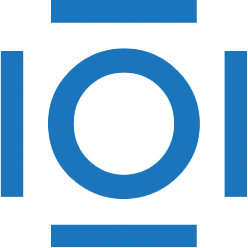
CITATIONS
Cited by 5 scholarly publications.
Image segmentation
Skin
Melanoma
Feature extraction
Image filtering
Photography
Image classification