Tumor volume is a topic of interest for the prognostic assessment, treatment response evaluation, and staging of malignant pleural mesothelioma. Many mesothelioma patients present with, or develop, pleural fluid, which may complicate the segmentation of this disease. Deep convolutional neural networks (CNNs) of the two-dimensional U-Net architecture were trained for segmentation of tumor in the left and right hemithoraces, with the networks initialized through layers pretrained on ImageNet. Networks were trained on a dataset of 5230 axial sections from 154 CT scans of 126 mesothelioma patients. A test set of 94 CT sections from 34 patients, who all presented with both tumor and pleural effusion, in addition to a more general test set of 130 CT sections from 43 patients, were used to evaluate segmentation performance of the deep CNNs. The Dice similarity coefficient (DSC), average Hausdorff distance, and bias in predicted tumor area were calculated through comparisons with radiologist-provided tumor segmentations on the test sets. The present method achieved a median DSC of 0.690 on the tumor and effusion test set and achieved significantly higher performance on both test sets when compared with a previous deep learning-based segmentation method for mesothelioma. |
ACCESS THE FULL ARTICLE
No SPIE Account? Create one
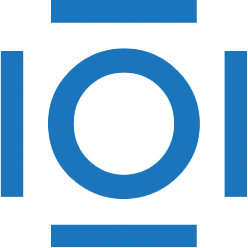
CITATIONS
Cited by 11 scholarly publications.
Tumors
Mesothelioma
Image segmentation
Computed tomography
Chest
Network architectures
Image processing