Purpose: Deep learning is a promising technique for spleen segmentation. Our study aims to validate the reproducibility of deep learning-based spleen volume estimation by performing spleen segmentation on clinically acquired computed tomography (CT) scans from patients with myeloproliferative neoplasms. Approach: As approved by the institutional review board, we obtained 138 de-identified abdominal CT scans. A sum of voxel volume on an expert annotator’s segmentations establishes the ground truth (estimation 1). We used our deep convolutional neural network (estimation 2) alongside traditional linear estimations (estimation 3 and 4) to estimate spleen volumes independently. Dice coefficient, Hausdorff distance, R2 coefficient, Pearson R coefficient, the absolute difference in volume, and the relative difference in volume were calculated for 2 to 4 against the ground truth to compare and assess methods’ performances. We re-labeled on scan–rescan on a subset of 40 studies to evaluate method reproducibility. Results: Calculated against the ground truth, the R2 coefficients for our method (estimation 2) and linear method (estimation 3 and 4) are 0.998, 0.954, and 0.973, respectively. The Pearson R coefficients for the estimations against the ground truth are 0.999, 0.963, and 0.978, respectively (paired t-tests produced p < 0.05 between 2 and 3, and 2 and 4). Conclusion: The deep convolutional neural network algorithm shows excellent potential in rendering more precise spleen volume estimations. Our computer-aided segmentation exhibits reasonable improvements in splenic volume estimation accuracy. |
ACCESS THE FULL ARTICLE
No SPIE Account? Create one
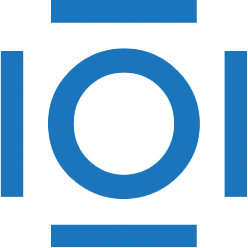
CITATIONS
Cited by 5 scholarly publications.
Spleen
Image segmentation
Computed tomography
Evolutionary algorithms
Convolutional neural networks
3D modeling
Image processing