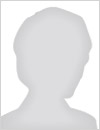
This will count as one of your downloads.
You will have access to both the presentation and article (if available).
In this paper, we will introduce a holistic approach for the pin accessibility scoring and routability analysis. For accessibility, the systematic calculator which assigns score for each pin will search the available access points, consider the surrounded router layers, basic design rule and allowed via geometry. Based on the score, the “bad” pins can be found and modified. On pin routability analysis, critical pin points (placing via on this point would lead to failed via insertion) will be searched out for either layout optimization guide or set as OBS for via insertion blocking. By using this pin routability and pin access analysis flow, we are able to improve the library quality and performance.
PB-DTCO flow can generate plenty of test patterns for model creation and yield gaining, classify candidate patterns systematically and furthermore build up bank includes pairs of match and optimization patterns quickly. Those banks can be used for hotspot fixing, layout optimization and also be referenced for the next technology node. Therefore, the combination of PB-DTCO flow with OPC not only benefits for reducing the time-to-market but also flexible and can be easily adapted to diversity OPC flow.
Using a pattern-based approach to detect all IP Blocks used provides the foundry advanced capabilities to analyze them further for any kind of changes which could void the OPC and process window optimizations. Having any changes in an IP Block could cause functionality changes or even failures. This also opens the foundry to legal and cost issues while at the same time forcing re-spins of the design.
In this publication, we discuss the methodology we have employed to avoid process issues and tape-out errors while at the same time reduce our manual work and improve the turnaround time. We are also able to use our pattern analysis to improve our OPC optimizations when modifications are encountered which have not been seen before.
This paper will present a working flow for using design diffing techniques to extract layout structures and perform a geometry analysis flow combined with testing results to find most probable suspects that may cause noticeable yield loss.
This paper will present a working flow for using pattern analysis interlayer profiling techniques to turn multiple layer physical info into group linked parameter values. Using this data analysis flow combined with an electrical model allows us to find critical regions on a layout for yield learning.
This paper will present a working flow for using design analysis techniques combined with diagnostic methods to systematically transform silicon testing information into physical layout information. A new set of the testing results are received from a new lot of wafers for the same product. We can then correlate all the diagnostic results from different periods of time to check which blocks or nets have been highlighted or stop occurring on the failure reports in order to monitor process changes which impact the yield. The design characteristic analysis flow is also implemented to find 1) the block connections on a design that have failed electrical test or 2) frequently used cells that been highlighted multiple times.
This paper will present a novel method of how to generate a complete profile of components for any particular design. The component checking flow need to be completed within hours so it will have very little impact on the tape-out time. A pre-layer checking method is also run to group commonly used layers for different electrical components and then employ different layout profiling flows. The foundry does this design chip analysis in order to find potentially weak devices due to their size or special size requirements for particular electrical components. The foundry can then take pre-emptive action to avoid yield loss or make an unnecessary mask for new incoming products before fab processing starts.
At advanced nodes, definition of design rules and process options must be tightly optimized to deliver the best tradeoff performance, power, area and manufacturability. However, implementation platforms don’t typically have access to process information and process teams don’t have design knowledge, and optimization loops required for Design-Technology-Co-Optimization (DTCO) are either impossible or at best long and expensive for fabless design house.
Joining forces, ASML, IMEC and Cadence Design Systems developed an In-design and signoff lithography physical analysis well suited for 7/5nm and below. The Tachyon OPC+ engine used by IMEC 7/5nm process has been integrated in Cadence Litho Physical Analyzer (LPA) to perform lithography checks using the foundry process models, recipes, and hotspot detectors. This flow leverages existing LPA infrastructure for both custom and digital design platforms, as well as standalone signoff.
Depending upon the end application, LPA could be launched either from place & route or custom layout or standalone. LPA processes first the design database to identify hierarchy, decompose the layout for coloring and apply pattern matching to identify location requiring simulation. The layout is then passed to the Tachyon OPC tool to perform optical process correction and model-based litho verification that is validated on Silicon. The hotspots and contours are processed by LPA for generation of hotspot marker and fixing guidelines and provide all this information to the design environment.
The flow has been developed and demonstrated to work on IMEC 7nm, and can be ported to smaller or larger technologies. The paper will present the result of this In-design and signoff lithography physical analysis flow, how DTCO and design teams can add manufacturability to PPA.
A new random layout generating method, Design Technology Co-Optimization Pattern Generator (DTCO-PG), is reported in this paper to create cell-based design. DTCO-PG also includes how to characterize the randomness and fuzziness, so that it is able to build up the machine learning scheme which model could be trained by previous results, and then it generates patterns never seen in a lite design. This methodology not only increases pattern diversity but also finds out potential hotspot preliminarily.
This paper also demonstrates an integrated flow from DTCO pattern generation to layout modification. Optical Proximity Correction, OPC and lithographic simulation is then applied to DTCO-PG design database to detect hotspots and then hotspots or weak points can be automatically fixed through the procedure or handled manually. This flow benefits the process evolution to have a faster development cycle time, more complexity pattern design, higher probability to find out potential hotspots in early stage, and a more holistic yield ramping operation.
This paper will present a smart and efficient working flow that can map inspection data back onto a design and produce more diverse monitor points for inspection, and each set of monitor points links to a set of statistical design data that shows insight on design structures that are more sensitive to the process variations. A full-chip post-processing flow is also implemented to process design layout so that the particular patterns that may cause certain function blocks to fail can be directly checked on post-processed layout.
This paper will present a novel methodology to enumerate initial test patterns based on other technology node products. With this novel methodology, DRM development and process capability verification can be sped up rapidly in comparison to a more traditional way. At the same time, the process weak-point signatures can be migrated from the older technology nodes to the new technology node for verification. This methodology will help foundries catch process detractor patterns at new technology early development stage.
For debugging silicon failures, DFT diagnostics can identify which nets or cells caused the yield loss. But normally, a long time period is needed with many resources to identify which failures are due to one common layout pattern or structure. This paper will present a new yield diagnostic flow, based on preliminary EFA results, to show how pattern analysis can more efficiently detect pattern related systematic defects. Increased visibility on design pattern related failures also allows more precise yield loss estimation.
This paper presents a novel method of how to generate test key patterns which contain known problematic patterns as well as any constructs which designers could possibly draw based on current design rules. The enumerated test key patterns will contain the most critical design structures which are allowed by any particular design rule. A layout profiling method is used to do design chip analysis in order to find potential weak points on new incoming products so fab can take preemptive action to avoid yield loss. It can be achieved by comparing different products and leveraging the knowledge learned from previous manufactured chips to find possible yield detractors.
In physical verification, DRCs represent dimensional constraints relating directly to process limitations. On the other hand, patterns represent the 2D placement of surrounding geometries that can introduce systematic process effects. It is possible to combine both DRCs and patterns in a single topological pattern representation. A topological pattern has two separate components: a bitmap representing the placement and alignment of polygon edges, and a vector of dimensional constraints. The topological pattern is unique and unambiguous; there is no code to write, and no two different ways to represent the same physical structure. Furthermore, markers aligned to the pattern can be generated to designate specific layout optimizations for improving manufacturability.
In this paper, we describe how to do systematic physical verification with just topological patterns. Common mappings between traditional design rules and topological pattern rules are presented. We describe techniques that can be used during the development of a topological rule deck such as: taking constraints defined on one rule, and systematically projecting it onto other related rules; systematically separating a single rule into two or more rules, when the single rule is not sufficient to capture manufacturability constraints; creating test layout which represents the corners of what is allowed, or not allowed by a rule; improving manufacturability by systematically changing certain patterns; and quantifying how a design uses design rules. Performance of topological pattern search is demonstrated to be production full-chip capable.
Using pattern classification technology and a relational database, GLOBALFOUNDRIES has constructed a pattern database (PDB) of more than one million potential yield detractor patterns. In PDB, 2D geometries are clustered based on similarity criteria, such as radius and edge tolerance. Each cluster is assigned a representative pattern and a unique identifier (ID). This ID is then used as a persistent reference for linking together information such as the failure mechanism of the patterns, the process condition where the pattern is likely to fail and the number of occurrences of the pattern in a design. Patterns and their associated information are used to populate DRC Plus pattern matching libraries for design-for-manufacturing (DFM) insertion into the design flow for auto-fixing and physical verification. Patterns are used in a production-ready yield learning methodology to identify and score critical hotspot patterns. Patterns are also used to select sites for process monitoring in the fab.
In this paper, we describe the design of PDB, the methodology for identifying and analyzing patterns across multiple design and technology cycles, and the use of PDB to accelerate manufacturing process learning. One such analysis tracks the life cycle of a pattern from the first time it appears as a potential yield detractor until it is either fixed in the manufacturing process or stops appearing in design due to DFM techniques such as DRC Plus. Another such analysis systematically aggregates the results of a pattern to highlight potential yield detractors for further manufacturing process improvement.
View contact details
No SPIE Account? Create one